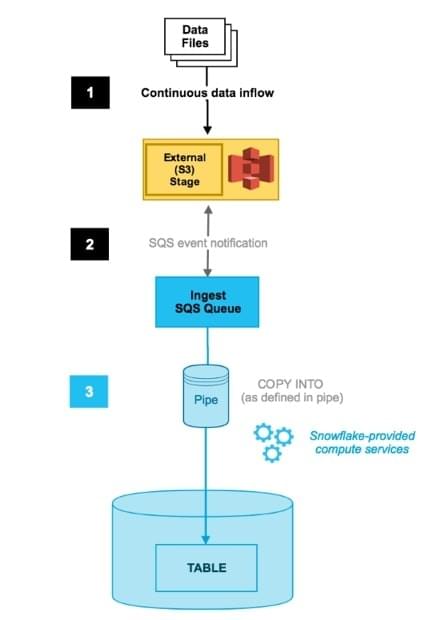
Snowflake Data Cloud supports the complete lifecycle of Machine Learning (ML) applications. This article describes set-based algorithms, and it also explains the use of Snowpark to move heavy custom processing into the Snowflake engine. Snowflake also has other features that make it more flexible. Read on to learn more about Snowpark and how it can help you improve your business intelligence. Using Snowflake for ML makes it easier to train your models and improve your business intelligence.
Dataiku's graphical interface makes it easy to load and analyze large datasets. Using Dataiku and Snowflake together helps you leverage the scalability of Snowflake while building more data products and deploying more projects in production. Together, Dataiku and Snowflake enable users to work on the same projects in a shared environment. They can use the same data without sacrificing performance.
As the data grows, users can collaborate on projects in both environments, including sharing their models and training them on the same dataset. With Snowflake, you can build ML models and create dashboards based on the data you've collected. You can also use the Snowflake UI to explore deeper into your data and create dashboards based on the ML predictions. Whether you're using traditional data science tools or you'd like to build your data science applications, Snowflake will help you get started. With Snowflake's connectors, you can build models and connect them to external data sources.Another great advantage of Snowflake Machine Learning is that it predicts demand and makes accurate recommendations based on various factors. This data can be collected from various sources, including weather, attendance, sales history, and item popularity.
It even uses weather data to predict travel dates. It can also predict future behavior. In addition to using the data you collect, Snowflake has a pre-built connector for SageMaker Autopilot. It provides data visualization, predictive analytics, and actionable AI.Dataiku enables you to push data processing tasks to Snowflake and take advantage of its full capabilities. Dataiku also provides in-database charting capabilities to deploy machine learning models into production. Dataiku's intuitive graphical interface lets you design data transformations and deploy them easily.
With Snowflake, you can get started with machine learning today. So, what are you waiting for? Make your data-driven decision-making a smoother process by using Snowflake. For training ML models, it is important to have a reliable process. One of the major problems with this process is lost data. Snowflake's time travel features can help you prevent lost data. The time travel feature does not support all use cases, but it saves you headaches when prototyping your ML model. You can use this feature for demand forecasting, demand prediction, and proof of concept projects. There is also a free 30-day trial, which can save you a lot of time. Snowflake is also capable of supporting Java user-defined functions (UDFs), which allow you to write custom logic in the model. ML-related UDFs can also be used with Snowflake, which makes them easier to write in Python. As of this writing, Snowflake's support for Python will greatly expand the possibilities for machine learning models in UDFs. For example, in one example, a user can use the ElectrifAi machine learning model to enrich Snowflake data, which is then deployed as a Snowflake UDF. This model will then output to the AWS Quicksight Dashboard. Check out this post for more details related to this article: https://en.wikipedia.org/wiki/Snowflake.